Telco AI Deep Dive: From cloud-native to AI-native
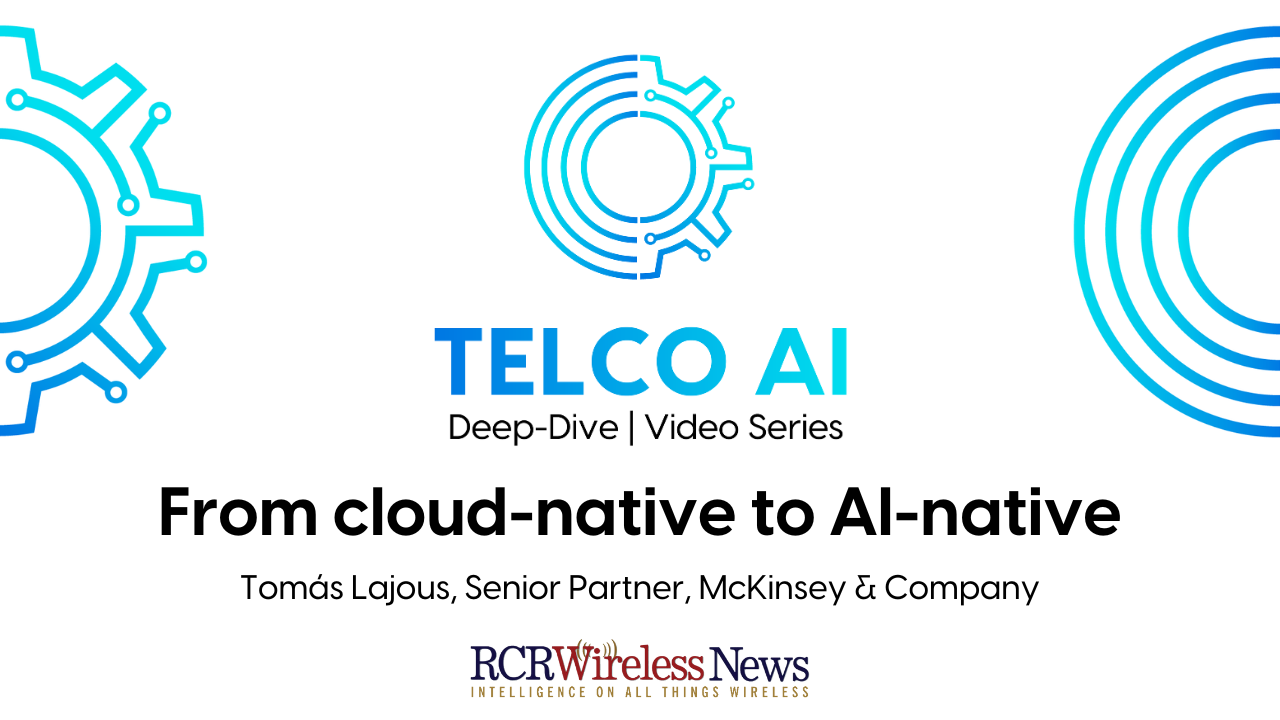
In the sweep of everything that could be as construed as “tech,” artificial intelligence (AI) is leading the current hype cycle with visions of broad productivity gains enabled by cloud computing, large language models (LLMs), natural language processing-based user interfaces and text/image generation capabilities. Telecommunications operators are no exception, shifting messaging to focus on AI for internal optimizations and customer-facing use cases that could generate new service revenues. Also worth noting is that although 6G is years away from standardization, early cuts at describing the next generation of cellular have honed in on the descriptor “AI-native.” But what does that really mean?
“Being cloud-native is a requirement for being AI-native
Last year McKinsey and Company published a report titled, “The AI-native telco: Radical transformation to thrive in turbulent times.” To better understand the vision and practical steps it would take to make that vision reality, RCR Wireless News talked with McKinsey and Company Senior Partner Tomás Lajous, a report co-author; Lajous acknowledged that being cloud-native is a requirement for being AI-native and that today operators have yet to become cloud-native.
“The notion of having a cloud-native telco, I think, is part and parcel to having an AI-native telco,” Lajous said. “And I think it’s worth it to just anchor a little bit on how we came to this notion of AI-native. Which is to say, if we were to start a telecom company from scratch today, what would be the best way to put it together? And that’s where we landed on the best way to put it together is by having AI at the core. And that means having AI assist essentially every decision and operating model, and a culture that embraces AI in order to do so, all the way from marketing and call centers to the network.”
Doing that, he said, means “you need very deep technical architecture that goes with it. And the best way to do [that] is by bringing in the cloud. And so you do require cloud for the AI part of it.”
Back to this idea of starting a telecom company “from scratch”—that’s not a luxury available to many and even modern cloud-native greenfield network builds like those undertaken by Dish in the U.S. and Rakuten Mobile in Japan have not translated into business success. Even so, the idea of becoming AI-native isn’t really a prescribed future state, Lajous said, rather it’s a “concept of how they need to be thinking about the future.”
He continued by describing elements that operators need to put in place: modernization of network technologies in all domains, the move to 5G Standalone core, and OSS/BSS upgrades. That last one relates to actually provisioning, charging and consuming services on the cloud-native network. As to how this relates to AI as an enabler of data monetization, Lajous acknowledged that regulatory constraints, user opt-ins and other factors have kept operators from fully leveraging the highly personal, contextualized data available to them.
Using AI in order to use more AI with the end goal of “making the product better”
But, Lajous said, “What I would highlight is that it’s not just for the traditional or the historical uses of profiling and marketing…Fundamentally it’s about making the product better. One of the issues that we’ve had in telecom is that it’s been very hard to assess the experience of customers, let’s say in a mobile network…Where AI comes in, is that now we can use AI to understand everything that’s happening on the network and understand relative to individual needs whether the experience is there or not.”
With tha information, he said, operators can improve the product, the customer experience and, from there, bring in additional differentiation. Another issue here is the availability of data within operator organizations; to be useful to an AI tool, data needs to be unified, organized and made available. As with many enterprises, operators are not immune from fragmented data sets locked into different silos.
“In the telecom space, we’ve been suffering with a vicious cycle of bad data leading to bad or insufficient AI, leading to less focus on generating data, leading to bad, insufficient data, and so forth,” Lajous said. “But we are breaking out of it. And there’s two elements to break out of it. The first one is the use of digital twins as the foundation for the AI strategies as opposed to use case-specific datasets…The second thing that I would highlight is gen AI…Gen AI actually helps meets that challenge.” He gave the example of circuit inventories and associated (voluminous) documentation. “Gen AI actually allow sus to grab all of that, put it together, and maybe not a system of record, but in a data product or a digital twin that can then be used to apply AI modeling to circuit data. And that’s one example how we can actually, with digital twins and gen AI, bridge that gap.”
Comments are closed.