What happens at the edge (should) stay at the edge
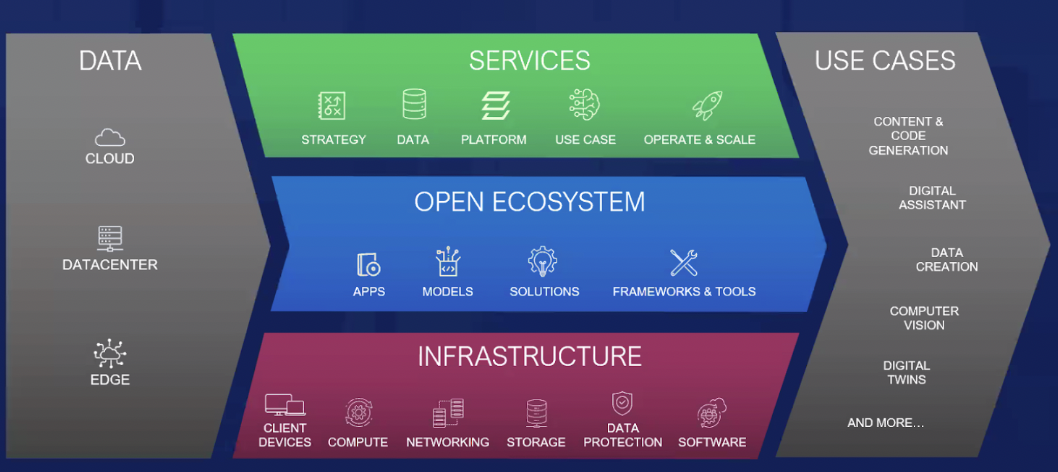
LAS VEGAS—Here at Dell Technologies World, there’s crystal clear acknowledgement that AI will be a very material force multiplier as essentially all companies endeavor to become technology companies. There’s also clear acknowledgement that doing AI isn’t as simple as writing a check to a vendor or vendors. The big challenges are around cost, complexity and risk associated with standing up a solution, aligning it with a high-value use case, demonstrating return on investment, then scaling that from one use case to many use cases. But before you even start down that path, there’s the data of it all.
“The world of computing is extending out to the physical world. The place where they meet is called the edge. A lot is going on there now. Much more will be happening soon.”
The bolded text above, and below, is from Michael Dell’s 2021 book Play Nice But Win. With its approach to building an AI factory, not to mention how it’s playing nice with NVIDIA, Servicenow, Microsoft and others, Dell Technologies sure looks poised to win a big piece of the AI pie. But another aspect of winning is that your customers also win. And before the troops are mustered around the necessary infrastructure, ecosystems and services, it starts with the data.
So step one, upstream of any AI implementation, is data hygiene; to deliver value, AI needs the right data—data from your company, data from the domain your company operates within, and (potentially) some of the data that’s fed in the hyped large language models (LLMs) that scrape the public internet to create that sort of non-linearity we see with other types of complex systems. To say that another way, garbage in, garbage out; or, more optimistically, gold in, gold out.
To the LLM piece, Dell Technologies Senior Consultant Nick Brackney told us that aspiring AI users need to dispense with the notion that bigger models are necessarily better models. “We’re in the process of educating them,” he said. “I basically say, ‘Do as little work as possible’…If you have a really clean data set, and you have a narrow model that’s really targeted at what you’re trying to solve for, that’ll perform better than” throwing the collected digitized knowledge of humanity at AI-enabled, computer vision-based quality assurance for a manufacturing firm, for instance. “We’re definitely seeing a lot of interest in smaller models.”
Brackney borrowed language from the 2000s era advertising push trying to drive home the point that we all have a role to play in environmental stewardship: reduce, reuse, recycle. In the context of AI, that means leaning into the idea of reducing the size of models; it means reusing models in support of different use cases; and it means recycling learnings, strategies and otherwise replicating what works.
Back to the edge: Dell’s COO Jeff Clarke threw out a figure during a Q&A session with media and analysts. He said that 83% of data is already at the edge. Given that the edge is the center of data gravity, the path forward is to bring the AI to the data rather than taking on the unnecessary complexity and cost of moving the data to the AI. This is part of a larger conversation around cloud repatriation the (re)rise of on-prem cloud.
“5G is especially thrilling—from connecting people to connecting things…it’s not about talking on the phone faster; it’s about making everything in the world intelligent and connected…All those things…will generate almost incomprehensibly massive amounts of data.”
In his book, Michael Dell picked the adjective incomprehensible to describe the volume of data that’s largely generated at the edge. Grounding it in the world of telecoms, Dell Tech’s Douglas Lieberman described operators’ ability to collect “ludicrous amounts of data. So they could build a powerful model very quickly…then you use it over and over and over again.” The low-hanging fruit here, and what we’re already seeing operators do, is use generative AI for call center/helpdesk-type applications. The next steps would, generally speaking, be to then focus efforts on opex reducing network optimizations and automations, then focusing on leveraging AI distributed throughout the network to drive new revenues—something like bundling connectivity and AI as a service in a way that makes better use of operators already massively distributed footprint. Seems like a no brainer.
But, Lieberman said, “I don’t think it’s any surprise that telcos move very slowly.” That’s attributable to a few things, chief among them regulatory machinations and what I’d describe as siloed organizational structures that are very much subject to inertia. This gets into the acknowledgement that telcos needs to reinvent themselves into techcos before they find themselves in existential territory. His take was that as soon as a pioneering player starts realizing material net-new value at scale, fast following will ramp “pretty quickly.”
And he was convincingly optimistic, calling the rise of AI “an inflection point in the industry.” Lieberman’s observation was that, based on 30-years in the industry, he’s never witnessed companies buying first and figuring out later. And, he said, this one isn’t going to be a passing fad that gets hung up in limited proofs and concepts and lab trials. Why? “Because the results are so instantaneous…accurate and useful. I think that you’re going to see adoption much, much faster. [And]…if you don’t do it, someone else is.”
And this gets back to Dell’s core value proposition which certainly includes AI but is also applicable across its portfolio; Michael Dell, talking about the AI Factory approach, called it an “easy button.” Lieberman echoed this sentiment. “Telcos are going to implement AI,” he said. “Unequivocally it will happen…We want to make it easy.”
Comments are closed.